Effect of Duration and Delay on the Identifiability of VR Motion
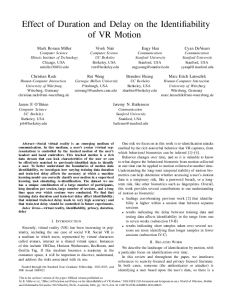
Download Paper
Download Bibtex
Authors
- Mark Miller
- Vivek Nair
- Eugy Han
- Cyan DeVeaux
- Christian Rack
- Rui Wang
- Brandon Huang
- Marc Latoschik
- James F. O'Brien
- Jeremy N. Bailenson
Related Links
Abstract
Social virtual reality is an emerging medium of communication. In this medium, a user's avatar (virtual representation) is controlled by the tracked motion of the user's headset and hand controllers. This tracked motion is a rich data stream that can leak characteristics of the user or can be effectively matched to previously-identified data to identify a user. To better understand the boundaries of motion data identifiability, we investigate how varying training data duration and train-test delay affects the accuracy at which a machine learning model can correctly classify user motion in a supervised learning task simulating re-identification. The dataset we use has a unique combination of a large number of participants, long duration per session, large number of sessions, and a long time span over which sessions were conducted. We find that training data duration and train-test delay affect identifiability; that minimal train-test delay leads to very high accuracy; and that train-test delay should be controlled in future experiments.
Citation
Mark Roman Miller, Vivek Nair, Eugy Han, Cyan DeVeaux, Christian Rack, Rui Wang, Brandon Huang, Marc Erich Latoschik, James F. O'Brien, and Jeremy N. Bailenson. "Effect of Duration and Delay on the Identifiability of VR Motion". In 2024 IEEE 25th International Symposium on a World of Wireless, Mobile and Multimedia Networks (WoWMoM), pages 70–75. IEEE Computer Society, June 2024. Presented at International Workshop on Privacy and Security in Augmented, Virtual, and eXtended Realities.